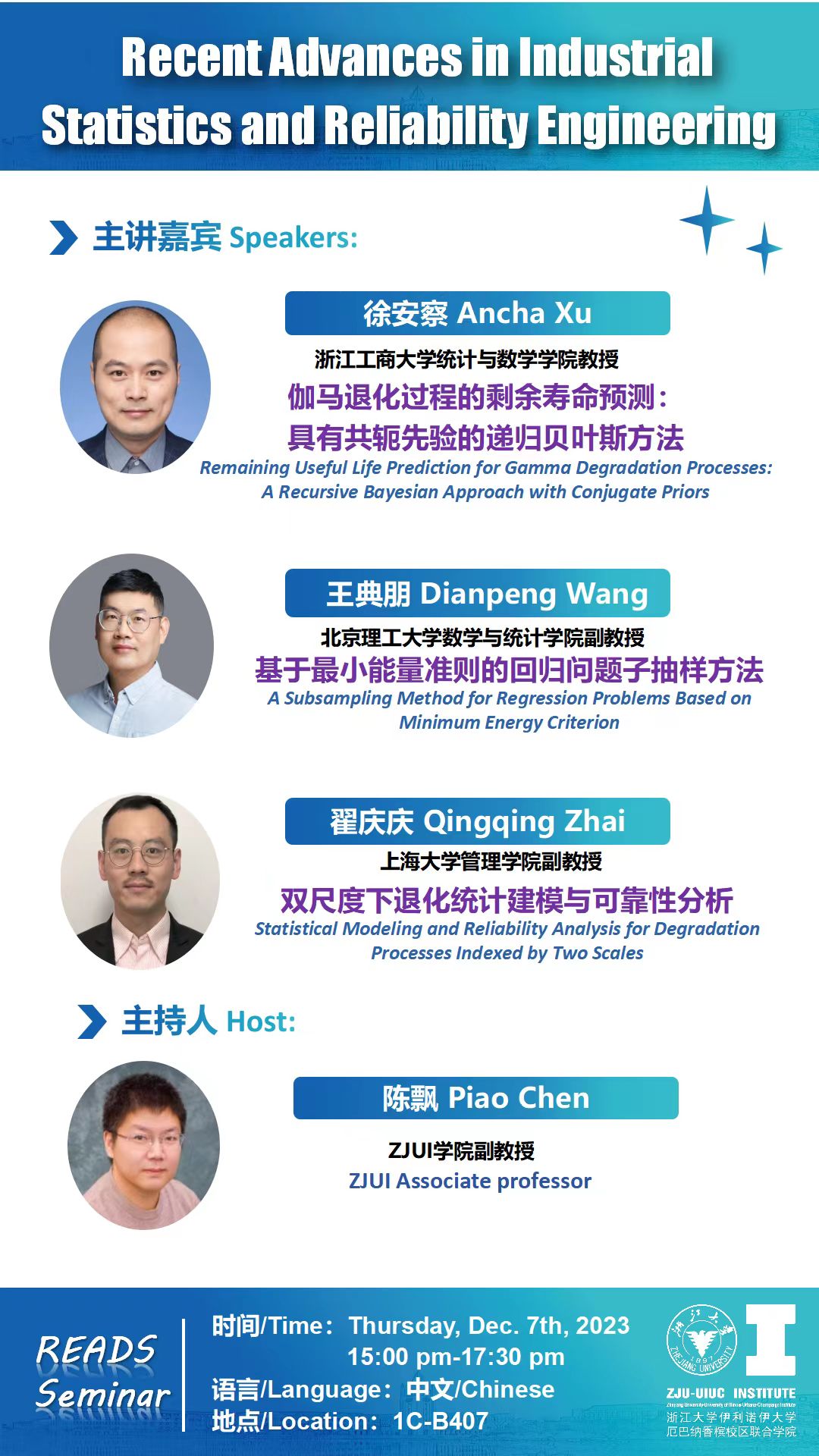
时间:12月7日(周四)15:00-17:30
语言:中文
Title: Remaining Useful Life Prediction for Gamma Degradation Processes: A Recursive Bayesian Approach with Conjugate Priors
Abstract: The Gamma process stands as a prevalent model for monotonic degradation data. However, its statistical inference faces complexity due to the intricate parameter structure within the likelihood function. This paper addresses this challenge by deriving a conjugate prior specifically for the homogeneous gamma process, investigating the properties of this prior distribution. To facilitate posterior inference, three meticulously designed algorithms (Gibbs sampling, discrete grid sampling, and sampling importance resampling) are employed to generate posterior samples of the model parameters. Through extensive simulation studies, these algorithms demonstrate notably high computational efficiency and precise estimation. Extending the conjugate prior to encompass the gamma process with heterogeneous effects, this study enables recursive updates to the posterior distribution of parameters. An innovative online algorithm is consequently developed, empowering the prediction of remaining useful life for multiple systems. The effectiveness of this online algorithm is demonstrated through comprehensive illustrations from two real-world cases.
Bio:徐安察,浙江工商大学统计与数学学院,教授,博士生导师,“浙江省高校领军人才培养计划”高层次拔尖人才,浙江省高校中青年学科带头人。担任第十一届中国运筹学会可靠性分会副理事长,中国现场统计研究会可靠性工程分会常务理事。主要研究领域为退化数据分析与建模、贝叶斯在线学习、寿命数据分析等。先后主持国家自然科学基金面上项目2项,青年项目1项,省部级项目5项,获第一届全国统计科技进步奖三等奖。
Title:A Subsampling Method for Regression Problems Based on Minimum Energy Criterion
Abstract:The extraordinary amounts of data generated nowadays pose heavy demands on computational resources and time, which hinders the implementation of various statistical methods. An efficient and popular strategy of downsizing data volumes and thus alleviating these challenges is subsampling. However, the existing methods either rely on specific assumptions for the underlying models or acquire partial information from the available data. For regression problems, we propose a novel approach, termed adaptive subsampling with the minimum energy criterion (ASMEC). The proposed method requires no explicit model assumptions and “smartly” incorporates information on covariates and responses. ASMEC subsamples possess two desirable properties: space-fillingness and spatial adaptiveness. We investigate the limiting distribution of ASMEC subsamples and their theoretical properties under the smoothing spline regression model. The effectiveness and robustness of the ASMEC approach are also supported by a variety of synthetic examples and two real-life examples.
Bio:王典朋,北京理工大学特别副研究员,博士生导师。王典朋副研究员在北京理工大学获得博士学位,中国科学院数学与系统科学研究院博士后,曾先后访问佐治亚理工、香港科技大学,担任北京大数据协会常务理事、中国现场统计研究会试验设计分会理事。主要从事敏感性试验设计、计算机试验设计、贝叶斯计算、等方向的研究。主持国家自然科学基金青年基金、面上项目和国家国防科技工业局先进星箭共性技术等项目多项,在Technometrics、Journal of Quality Technology、Statistica Sinica、RESS、Applied Mathematical Modelling等统计学权威期刊上发表论文多篇。
Title:Statistical Modeling and Reliability Analysis for Degradation Processes Indexed by Two Scales
Abstract:Degradation is an important phenomenon for industrial products, which manifests as the gradually deterioration of some performance characteristics. The degradation process is often relevant to both time and usage, and indexing the degradation process merely by the time or usage cannot characterize the process accurately. Considering a stochastic usage process, this study proposes a degradation process model indexed by two scales, i.e., the time and the usage, where the degradation along the two scales are modeled as correlated nonlinear Wiener processes. We develop two simulation-based algorithms for reliability evaluation and study the model inference problems for the proposed model. The estimation procedure and the reliability assessment algorithms are validated by simulations. The performance of the proposed model is justified with an application to a real degradation dataset of outdoor coating materials, which shows that indexing the degradation process by two scales can considerably improve the degradation modeling performance.
Bio:翟庆庆,上海大学管理学院副教授,上海市青年东方学者。担任中国现场统计研究会可靠性工程分会副秘书长,中国双法学会工业工程分会理事,中国系统工程学会系统可靠性工程专委会委员。2015年于北京航空航天大学系统工程专业获得博士学位,2015至2017年在新加坡国立大学工业系统工程与管理系担任research fellow. 主要研究兴趣包括退化统计模型、可靠性建模等。在Technometrics、IISE Transacti ons、ITII、EJOR、ITR、RESS等国际期刊上发表论文40余篇。先后主持国家自然科学基金面上项目1项,青年项目1项。