Recently, research findings by Assist Prof. Zhang Qiang of ZJUI, titled "Knowledge aware Reinforced Language Models for Protein Directed Evolution," was accepted by the top conference in the field of machine learning, ICML 2024. This work constructed a Knowledge Aware Reinforcement Language Model (KnowRLM) that can effectively identify high fitness mutants and find the optimal pathway for amino acid transformation through an amino acid knowledge graph. The co-first author and corresponding author of this paper is Assist Prof. Zhang Qiang, of ZJUI.
Proteins are key molecules in organisms that perform various functions, and scientists have long been committed to optimizing protein function through directed evolution. Directed evolution is a process that simulates natural selection by mutating and screening proteins to discover variants with better performance. However, traditional directed evolution methods have problems such as low efficiency and limited mutation screening range. In recent years, scientists have started using machine learning (ML) to accelerate this process, known as machine learning assisted directed evolution (MLDE). However, these methods often only focus on the data itself and overlook the valuable knowledge accumulated by biologists, such as the complex biochemical relationships between amino acids.
In order to address the above issues, this paper proposes a new method called Knowledge Aware Reinforcement Language Model (KnowRLM), which combines biological knowledge and reinforcement learning algorithms to more accurately guide the mutation process of proteins, significantly improving the efficiency of protein directed evolution.
1 About the Article
In this study, researchers proposed an optimization framework based on reinforcement learning, which combines the statistical characteristics of protein sequences and the biochemical properties of amino acids to optimize the mutation process of proteins. The research group has constructed an Amino Acid Knowledge Graph to capture the complex associations between amino acids, enabling the knowledge aware reinforcement language model to better understand the structure and function of proteins and provide more guiding references for mutations.
Combining knowledge graph, this method first uses the Protein Language Model for mutation prediction. During the mutation process, the system evaluates the mutation effect of each step through reinforcement learning strategies, provides feedback for knowledge perception strategies, and adjusts the mutation path based on the feedback results, gradually optimizing the fitness (i.e. functional performance) of protein sequences. This method effectively overcomes the limitation of traditional random mutations that can only achieve local optima. Through continuous learning and adjustment, a globally optimal mutation scheme can be achieved.
This study demonstrates the enormous potential of introducing knowledge aware reinforcement learning into protein directed evolution. With the further integration of bioinformatics and artificial intelligence technology, this method has broad application prospects in fields such as drug development and industrial enzyme optimization. By continuously improving the predictive ability of AI models, it is expected to accelerate innovation in the field of biotechnology and promote the development of multiple industries such as precision medicine and green chemistry in the future.
▲ Schematic diagram of Knowledge Aware Reinforcement Language Model (KnowRLM)
2 About the Author
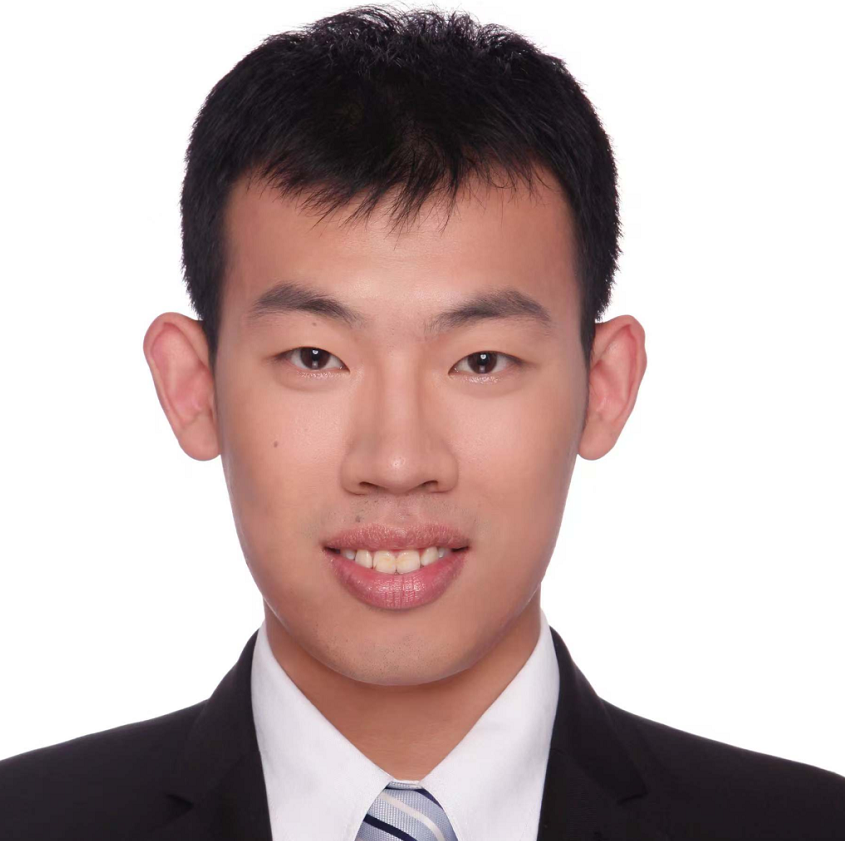
Dr. Qiang Zhang obtained his Ph.D. degree and served as a postdoctoral researcher, both at the Department of Computer Science, University College London in the United Kingdom. He was supervised by Prof. Emine Yilmaz, an internationally renowned expert in the field of information retrieval and natural language processing. He has published over forty articles in top-tier academic journals and conferences including Nature Machine Intelligence, Nature Communications, NeurIPS, ICML, ICLR, AAAI and ACL. He has numerous award such as the Great Britain-China Educational Trust in 2020.
Article Link:https://proceedings.mlr.press/v235/wang24cq.html