Recently, Assistant Professor QIAN Chao of ZJUI, together with domestic and international researchers, proposed deep learning enabled homeostatic neuro-metasurfaces to achieve realtimewireless channel management in dynamic environments. The results were published in Science Advances entitled “Homeostatic neuro-metasurfaces for dynamic wireless channel management”, with an impact factor of 14.957.
Smart city is a generic term used to describe an urban area that leverages information and communication technologies (ICTs) to optimize transportation systems, social sustainability, resource allocation and other community services. In particular, recent decades have witnessed an unprecedented promotion due to the big advances in the Internet of Things (IoT) and artificial intelligence. Much effort has been initiated to pursue intellectualization at the data link level and network level. However, the physical level—wireless channel, an electromagnetic (EM) link between the transmitter and the receiver with complex propagations inside—also plays an important role. If we can manage the wireless channel as desired, a radically new EM smart city/infrastructure could be created.
A fundamental backbone to create the aforementioned vision is to physically manage the wireless channel and modify the EM environment even in disordered surroundings. Conventionally, the engineering of wireless channel typically necessitates highcomplexity and energy-consuming hardware at base stations, and it is limited by lengthy and iterative optimization strategies. In the past years, metasurfaces—an artificial wave-functional interface composed of arrays of subwavelength resonators—have attracted extensive attention for manipulating a wireless channel in a green and cost-effective manner. However, the related metasurfaces based works share a common limitation: either they are static in nature (set in stone after fabrication) or work in a trial-and-error mode to satisfy user demands (dependent and iterative).
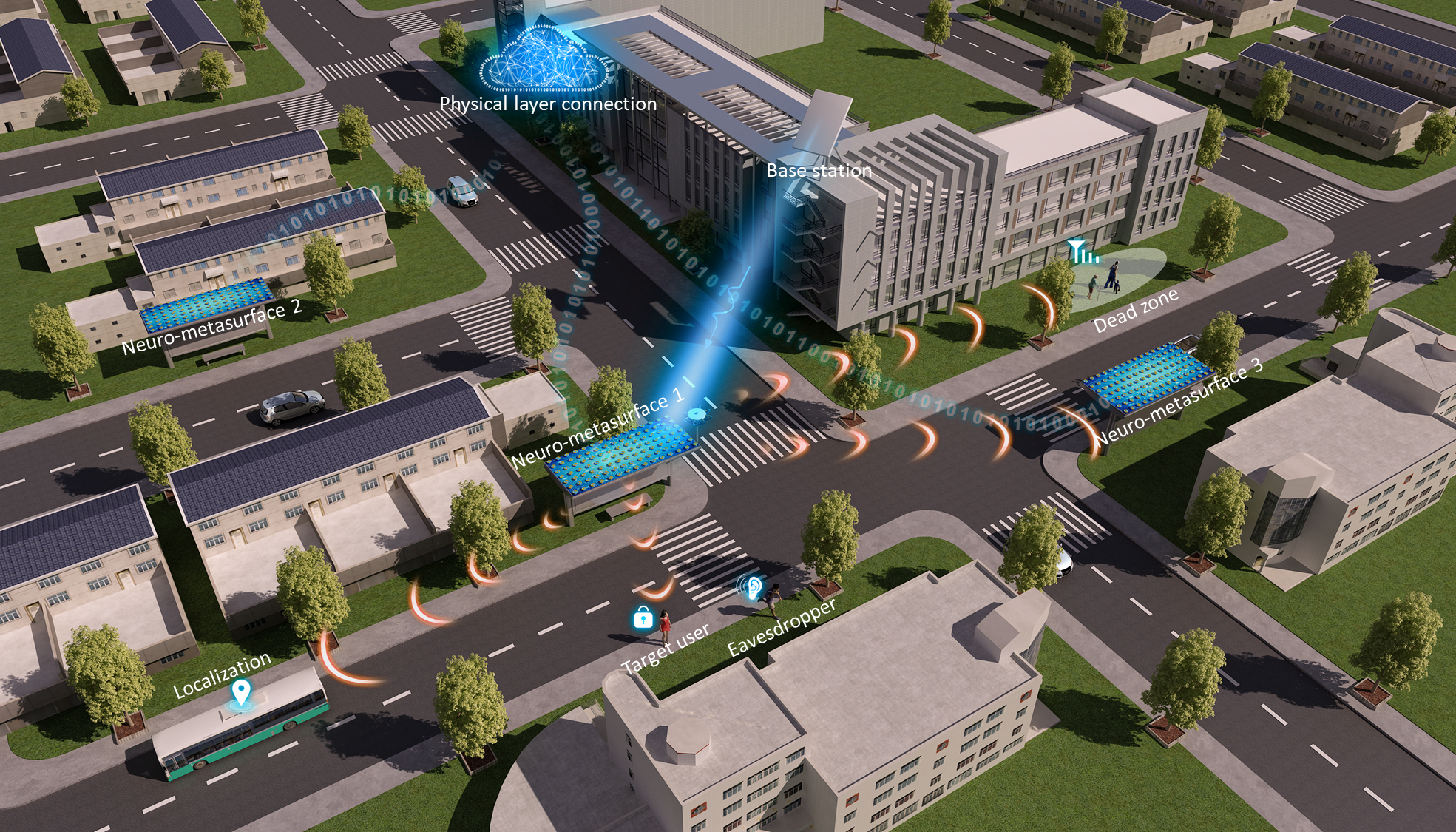
▲Figure 1. Schematic diagram of the application of Homeostatic neuro-metasurfaces in EM smart city
The group proposes the concept of homeostatic neurometasurfaces that manage the wireless channel globally and monolithically during the propagation process to automatically meet users’ requirements. The neuro-metasurfaces are composed of a large number of active metasurfaces units, where each active element modulates the amplitude, phase, and polarization of the incident wave independently. A miniature stepper motor applies mechanical drive to each neuro-metasurfaces unit. This mechanical modulation does not require a continuous energy supply and has the advantages of nonvolatility and low energy consumption, which improves the resistance of the metasurfaces to interference in an unstable environment. Based on the appropriate amount of training data and data augmentation techniques, a global inverse design model based on deep learning is established to build an “expressway” between the wireless channel and the arrangement of the neuro-metasurfaces.
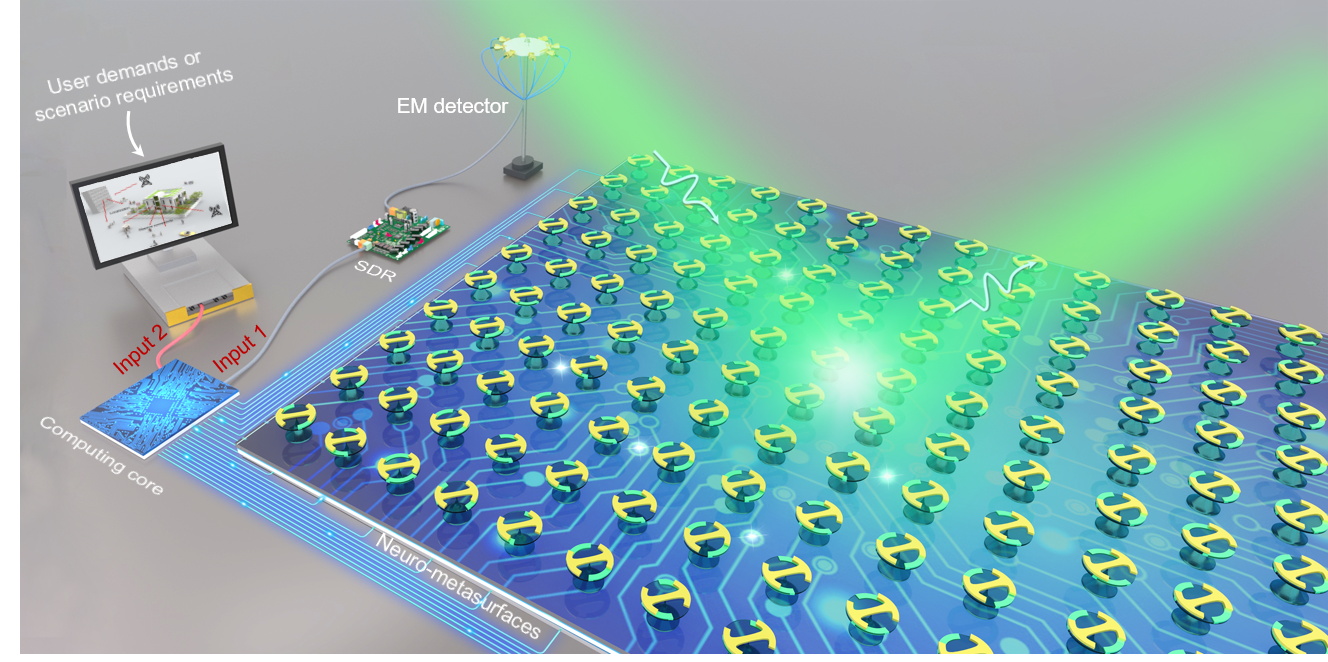
▲Figure 2. Perception (EM detector)-decision (global inverse design)-execution (neuro-metasurfaces) experimental system
The research team experimentally built a perception (EM detector)- decision (global inverse design) - execution (neuro-metasurfaces) system. The generality of global inverse design and the robustness of the neuro-metasurfaces were verified by first randomly selecting a test set and then extending the experimental environment to real scenarios to dynamically manage the wireless channels without human intervention.
This work combines metasurfaces with deep learning to empower neuro-metasurfaces the ability to independently analyze and solve problems, providing new ideas for EM smart city infrastructure construction. This on-site problem-solving capability is expected to be extended to areas with high real-time requirements, such as dynamic EM stealth and imaging in random media.
Dr. FAN Zhixiang is the first author of the paper, and Assistant Professor QIAN Chao is the corresponding author. The research was done in collaboration with Prof. CHEN Hongsheng, Prof. LI Erping, and Associate Prof. ZHENG Bin. The work was supported by the National Natural Science Foundation of China and other projects.
Article link: https://doi.org/10.1126/sciadv.abn7905